AI recap: The Rise of the Prompt Engineer and Biased Driverless Cars
"Explore the rise of the Prompt Engineer and challenges with biased driverless cars in this AI recap. Stay informed about AI trends."
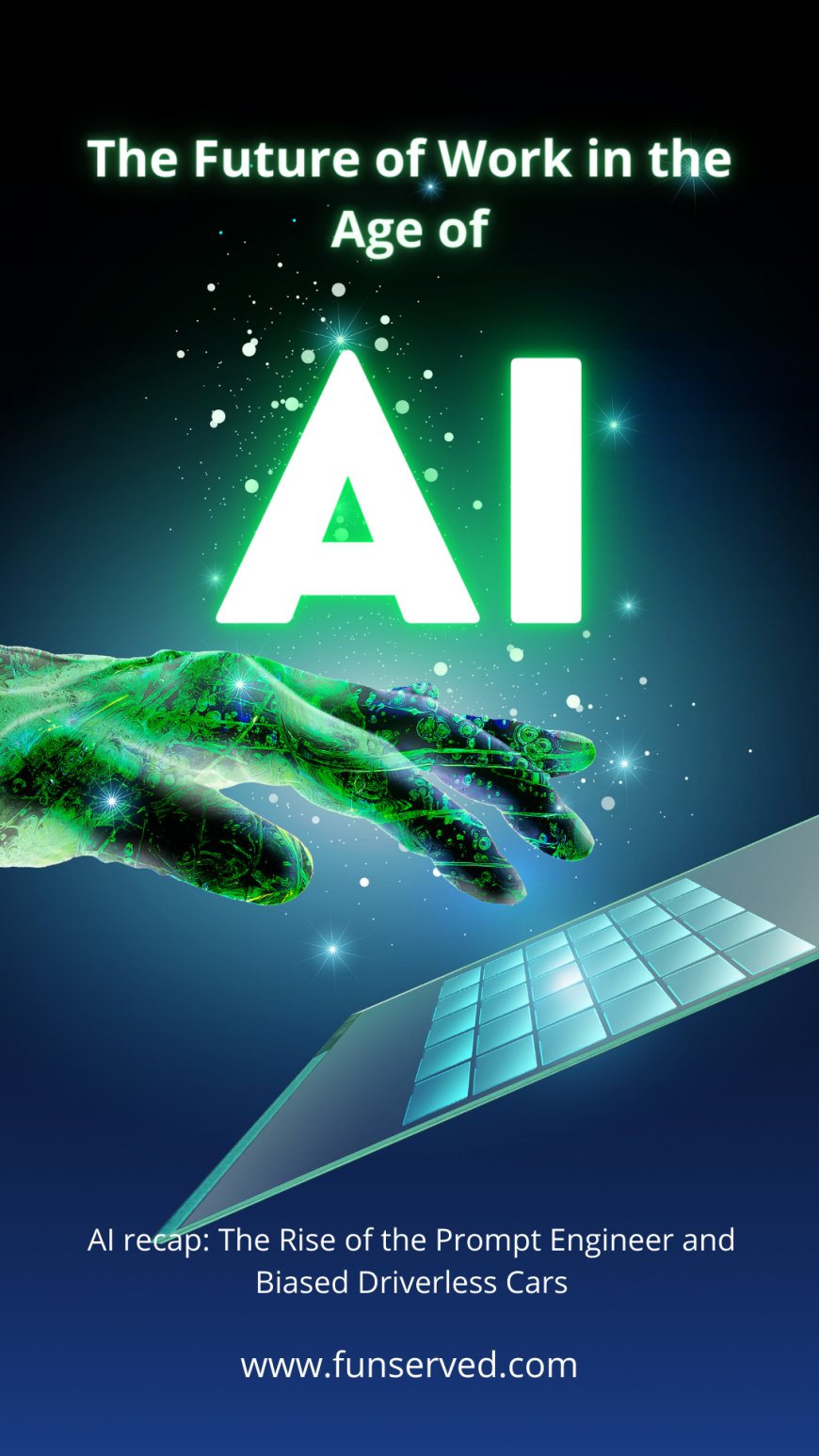
As humanity ventures deeper into the annals of the 21st-century zeitgeist, the technological watershed moments are encapsulated predominantly by the ascendancy of Artificial Intelligence (AI). Far transcending mere gadgetry and domiciliary automation, AI has metamorphosed into the quintessential chauffeur of our collective destiny—most tangibly illustrated by the advent of autonomous vehicular transportation. This oeuvre elucidates the underemphasized yet indispensable roles of prompt engineering and AI ethnocentrism amelioration in vehicular autonomy.
Quintessential Technological Apogees: AI Unveiled
Artificial Intelligence is no longer consigned to the annals of speculative fiction; it represents an apodictic actuality. Modalities such as machine learning, neural networks, and deep learning have transmuted into quotidian nomenclature. These algorithmic conduits imbibe voluminous datasets to engender cognitive faculties, culminating in self-navigating vehicular systems. Yet, the question that begs assiduous exploration is: how do we inoculate these systems against fallacious data interpretation?
Ethical and efficacy issues in AI-induced vehicular autonomy
In the realm of automated vehicles, the prompt stimuli ingested by AI and their subsequent analytical dissection can substantively dictate systemic performance. Given the gravitas of the human lives hanging in the balance, this is of unparalleled significance.
Prompt Engineers: The Arcane Alchemists of AI Ontology
Epistemological Deciphering of Prompt Engineering:
Prompt engineering epitomizes the art of finessing the interrogative stimuli so that AI taxonomies, such as GPT models, yield cogent and efficacious outputs. It's akin to navigating a cognitive labyrinth to ascertain a singular locus of veracity.
Prompt Engineers as Systemic Influencers:
These arcane manipulators of data queries mold the existential functionalities of AI models, ensuring concordance between analytic output and intended ramifications. Within vehicular autonomy, this translates into calibrating AI systems to differentiate an animate pedestrian from an inanimate lamppost while also cognitively deciphering variances in traffic semaphore interpretation.
The Dangers of AI Ethnocentrism in Vehicle Autonomy
Demystifying the Quagmire of AI Ethnocentrism:
AI ethnocentrism is an epiphenomenal manifestation of inherently skewed training paradigms. The data regurgitates the biases imbued within its foundational architecture, thus threatening to propagate the same.
Quintessential Risks in AI-Induced Autonomy:
The parochialism of AI, particularly when cemented by locale-specific data, can yield catastrophic repercussions, escalating from suboptimal decision-making to flagrant endangerment of human life.
Controlling Ethnocentric Malaise in Autonomous Vehicles
Ameliorating such bias necessitates the cultivation of a more ecumenical training dataset, nuanced scrutiny through iterative feedback loops, and a multidisciplinary consortium involving technologists, ethicists, and prompt engineers.
In sum, as AI catapults humanity into an odyssey filled with boundless prospects, the oracular challenges of prompt engineering and AI ethnocentrism mandate meticulous attention. Only through such scrupulous efforts can we hope to engender an era of autonomous vehicles that are not just efficacious but also ethically unimpeachable.
Techniques to Reduce Bias in AI Algorithms
Within the protean labyrinth of artificial intelligence (AI), bias abatement operates as an axiomatic prerogative. Emerging methodologies for this purpose entail an eclectic medley that transcends conventional paradigms. Adversarial training functions as an intricate chess match, pitting AI modules against virtual nemeses to unmask and redress prejudices in algorithmic cognition.
Counterfactual fairness employs statistical calibrations to neutralize latent confounders potentially exacerbating discriminatory outputs. Synthetic data generation serves to interpolate the epistemological vista of algorithms, concocting an expansive compendium of simulated experiential contexts that buttress decision-making fidelity.
Technicalities in Regulatory and Ethical Sanctums
Regulatory ingress into the realm of AI engenders a Gordian knot, interlacing legislative, ethical, and technocratic dimensions into an intricate tableau. Normative instruments like the Algorithmic Accountability Act endeavor to instantiate a regulatory bulwark, particularly imperative within the treacherous purview of autonomous navigation systems.
Concurrently, ethical considerations transcend mere legislative compliance, mandating an intertextual discourse that enfolds societal strata, cultural heterogeneity, and the sanctity of corporeal existence. A synergistic concert between multiple stakeholders—including ethicists, policy architects, and computational savants—is a sine qua non for fabricating a cohesively ethical schema for AI.
Ontological Evolution of AI Paradigms Effects
Ingrained bias serves not merely as a tarnishing stain on the algorithmic mirror but also as a circumscribing fetter, inhibiting the emancipatory trajectory of AI into vanguards of disruptive innovation. Discriminatory algorithms engender a cyclical perpetuation of systemic aberrations and imprecisions, triggering ethical firestorms and operational quandaries. Such limitations sap the foundational trust in AI, instigating disillusionment amongst consumer cohorts and sector-specific incumbents, thus thwarting the grandiloquent vision of ubiquitous AI integrations.
Bias-Induced Autonomous Vehicle Experiences:
Publicized tableau vivant of bias-driven mishaps in driverless automobiles have crescendoed into cacophonous uproars, spotlighting an exigency for enhanced scrutiny. For instance, an ostensible lacuna in Tesla's Autopilot algorithm to efficaciously discern complexions across a melanin spectrum invited strident critiques, amplifying demographic-specific risk indices. These instances serve as incendiary crucibles, intensifying the imperative to consecrate anti-bias strictures within algorithmic engineering matrixes.
Bias and Algorithmic Technology Epistemic Trust:
The dissemination of bias-infected algorithms catalyzes an existential schism in the relational dynamics between AI edifices and their prospective constituencies. When the public arena identifies AI frameworks as arborescent repositories of historical and systemic prejudices, the reverberations catalyze stifling inertia in the advancement and mass assimilation of AI paradigms.
The Future Lexicon of Prompt Engineering
Considerations for Prompt Engineering Expansions:
As AI imbues the quotidian fabric of human existence, prompt engineering undergoes an evolutionary ascent toward unprecedented significance. Leverage from nascent technological edifices like quantum computation and probabilistic syntactic platforms could potentiate a recondite sophistication in the art of calibrating machine-interpretable queries and directives.
Transcendental Effects on AI Applications:
The transcendental implications of prompt engineering breach the circumscribed confines of vehicular autonomy. Envision a revolution in personalized medicine, where algorithms, propelled by perspicaciously engineered prompts, deliver bespoke therapeutic regimens. Alternatively, ponder the legalistic matrices where AI, given suitably contextual prompts, could elucidate labyrinthine jurisprudential archives.
In summary, the artifice and perils surrounding AI, prompt engineering, and inherent biases constitute a multifaceted tapestry of interlocking challenges and opportunities, imploring a vigilance commensurate with their transformative potential.
Frequently Asked Questions
Q. What is algorithmic bias?
Algorithmic bias refers to the systemic anomalies in computational frameworks that generate discriminatory or unjust outcomes, often reflecting existing social prejudices. It is a perplexing issue that arises when an AI system's decision-making process becomes skewed due to prejudicial data or misaligned training objectives.
Q. Can bias be completely eliminated from AI?
The pursuit of completely eradicating bias in AI algorithms resembles the philosophical conundrum of Zeno’s paradox: one can continually halve the distance to the goal but never truly reach it. As AI systems are trained on data that inherently carry human biases, the elimination of bias becomes a Sisyphean task.
However, with vigilant engineering and ethical oversight, it is possible to substantially mitigate, if not entirely eradicate, these biases.
Q. How can consumers ensure the safety of AI-driven products?
Consumer safety concerns about AI-infused goods need a multifaceted solution. First, examine algorithmic development's parent company's ethics. Second, knowing the training datasets' data history and provenance might reveal bias. Finally, using third-party audits and validations to adopt a 'trust but verify' strategy may offer confidence.
Conclusion
The journey through the labyrinthine intricacies of AI and prompt engineering showcases a pantheon of concerns and methodologies. Prompt engineering emerges as a crucible in which the alloy of AI's responsiveness and utility is smelted, dictating how accurately and ethically an AI model responds to a plethora of scenarios. Algorithmic bias, on the other hand, manifests as a Gordian knot that engineers and ethicists must work tirelessly to untangle, given its ability to mar not just the functionality but the very fabric of social ethics within AI systems.
In the cosmic scale of technological evolution, AI is still in its infancy, teetering on the brink between its enormous potential and the perils that accompany it. Therefore, it is of quintessential importance that we deploy an indefatigable vigilance in its development and implementation. Both the ingenuity of prompt engineering and the meticulous deconstruction of bias are not mere adjuncts but core necessities in our collective AI odyssey.
What's Your Reaction?
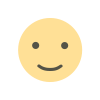
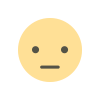
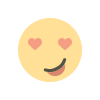
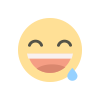
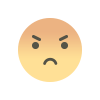
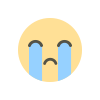
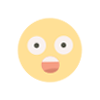